The guide discusses how an enterprise can use predictive maintenance AI.
In this article
- Traditional Maintenance Challenges
- What Is Predictive Maintenance?
- Predictive Maintenance AI to the Rescue
- Frequently Asked Questions on Predictive Maintenance
According to a study, unplanned downtime costs industrial manufacturers an estimated $50 billion each year. With this in mind, the importance of a facility’s maintenance strategy is hard to overestimate.
First let’s see what were the challenges in using the traditional maintenance procedure.
Traditional Maintenance Challenges
It‘s worth starting off with some of the challenges often associated with traditional maintenance or preventative maintenance processes. Traditional maintenance checks can be manual, time-consuming and be an inefficient use of manpower, and expensive if your business has thousands of components and/or machinery to test.
For example, a maintenance manager might have historical information that shows that a component is about to fail after running for 2000 hours. The maintenance manager might then schedule in a work order in a maintenance calendar to instruct employees to check this motor after it had been running for 2000 hours.
Planned maintenance like this might be ok if the maintenance manager is responsible for only a handful of components or equipment but this is never really the case and it‘s these sorts of challenges in mind that predictive maintenance sets out to resolve.
What Is Predictive Maintenance?
Predictive maintenance refers to the process of predicting when equipment might fail and performing maintenance in order to prevent the occurence of failure. Time series and historical failure data are used for such predictions.
Predictive Maintenance AI to the Rescue
A solution that leverages predictive maintenance can take real-time streamed data from the motor or component that contains operating levels, how many hours it’s been active, size, diameter or circumference and so on, then automatically check these against defined acceptable operating levels and surface any potential data points that suggest a fault is about to develop.
This brings us to our first topic regarding ways AI can help with predictive maintenance — pattern and anomaly detection.
Pattern and Anomaly Detection
One of the major benefits the enterprise can get from adopting a predictive maintenance solution that leverages artificial intelligence is the warning that a fault is about to develop. Couple this with the ability to detect optimal operating patterns for critical assets and it‘s easy to see why a predictive maintenance solution can be favored in contrast with a more traditional approach.
Get a complimentary discovery call and a free ballpark estimate for your project
Trusted by 100x of startups and companies like
One example in the real world where artificial intelligence could be used with predictive maintenance is in the oil and gas sector, or, to be more precise, oil rigs.
Consider a predictive maintenance implementation based on ML models that can identify patterns from historical datasets that contain training data labeled with “successfully running” components and “about to fail components”.
The machine learning model could ingest real-time sensor data signals from IoT devices attached throughout the rig, process this sensor data and then identify patterns or anomalies for a human to examine.
All this can take minutes as opposed to hours with traditional approaches and can, therefore, save the enterprise from unnecessary downtime, reduce maintenance costs, descrease repair cost, and ensure process or workflows have almost constant uptime.
The IoT (Internet of Things) value add
A key feature of pattern and anomaly detection involves the use of IoT devices, for example, an elevator manufacturer developed an IoT solution called MAX that is used for 110,000 elevators around the world.
MAX ingests door movement, power-up, trips, car calls and error codes and pushes this information to the cloud where it is then processed and crunched by complex machine learning algorithms to help spot patterns and likely failures.
By leveraging IoT connected devices and machine learning algorithms, MAX can predict maintenance issues and anomalies before they occur and help give elevator engineers advance warnings when an elevator may be due to become faulty, it can even suggest which components to replace, thereby making the elevator engineers job a little bit easier!
Learn about top IoT cloud platforms on our blog here.
This brings us to predictive repairs and how the enterprise can use artificial intelligence and predictive maintenance to help predict items that may need repair.
Predicting Repairs
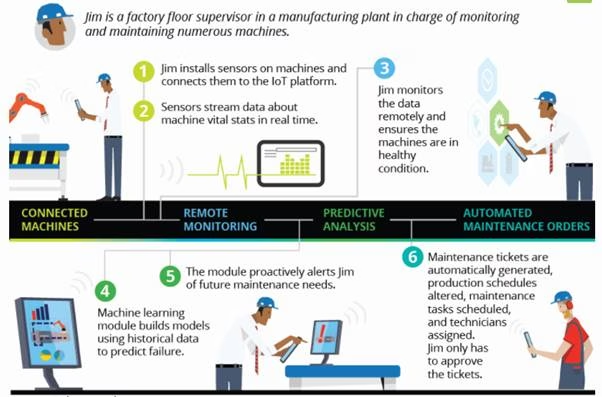
(Image courtesy of Deloitte)
One of the biggest value-adds that artificial intelligence and predictive maintenance can bring to the enterprise is that of being able to automatically predict future outcomes when maintenance checks should be performed, prior to equipment becoming faulty.
You can see in the image above how IoT devices are a crucial element of an AI-powered predictive maintenance solution and there are many more benefits.
A solution like this reduces the number of manual or planned maintenance checks that need to be performed, not to mention unnecessary downtime which ultimately saves costs on manpower and business downtime.
Our article discusses how AI can help with improving quality control procedures.
C3 AI
One good example of AI with predictive maintenance in action is in the C3 Predictive Maintenance solution. Some of the key features include, amongst other things are real-time asset monitoring, long-term risk assessment, and AI/machine learning-based risk scoring.
Hire expert developers for your next project
1,200 top developers
us since 2016
The product can be used in a variety of use cases, a major European integrated electric utility deployed the solution to help accurately identify and predict potential equipment failure in power plants.
It integrated live operational data where data refreshes nearly every 10 seconds as well as ingesting 4 years of historical data, all of which let users predict if failures were about to occur within up to 3 weeks‘ notice!
This deployment of the C3 AI solution is on target to reduce the cost of equipment failures by up to 100,000 euros per incident!
“Smart” factories of the future that deploy predictive maintenance solutions that leverage artificial intelligence and IoT devices are can also ingest data from multiple sources and/or legacy systems to provide real-time advanced analytics which brings us onto our next topic — analytics and insights.
Analytics and Insights
Ingesting data from IoT devices or multiple data sources can result in datasets that vary in terms of their frequency, structure, and size. All this information must be centralized and go through processing and parsing, cleansing, and storage.
It‘s these machine learning models that can help find “signals in the noise” as components or equipment are used throughout their daily operations.
Refined Failure Thresholds
For example, failure thresholds can initially be defined by humans based on real-world experience, machine learning technology can then, over time, gradually improve these thresholds by analyzing the outcome of each predictive insight.
Optimized Stock Levels
Having access to deeper insights such as anticipated failure dates/times of components can also help the enterprise optimize stock quantities.
Business no longer needs to overstock unnecessary parts as analytics, powered by intelligent machine learning algorithms can advise human operators when new orders should be made for components.
With this sort of automation and analytical insight, predictive maintenance solutions that leverage artificial intelligence can vastly reduce the cost of daily operations.
A key pillar of this, however, is choosing the right analytics solution and algorithms for the tasks at hand, that said, as machine learning algorithms enter the mainstream (with some now being offered as SaaS web service endpoints), this sort of technology is now within the reach of businesses and organisations that may not have employees with a Ph.D. in Computer Science.
Read more on how manufacturers can save huge resources through AI in various use cases.
Fleet and Asset Management
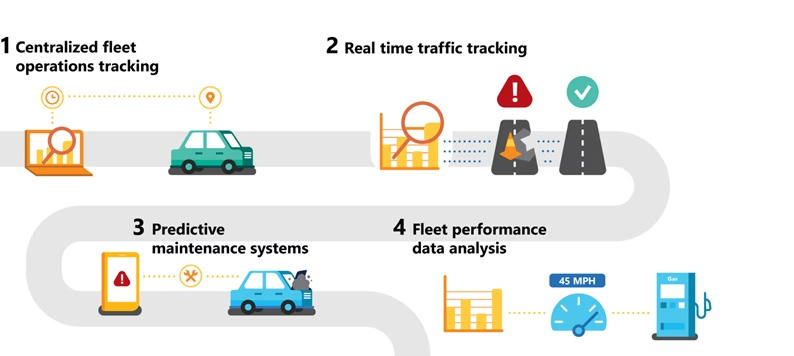
Predictive maintenance services and artificial intelligence are just reserved for production lines or manufacturing plants. For example, PdM can be applied to fleet and vehicle management, sensors can be attached to transportation ships and measure attributes such as temperature or pressure.
Human operators can then receive notifications in advance whether an engine component is on the verge of a breakdown. This can give employees valuable time to adjust course or get to work at replacing the component prior to the actual breakdown.
Other examples of predictive maintenance software can be applied to fleets of trucks, buses or cars to help prevent breakdowns before they even occur, thereby ensuring that delivery times are honored.
Blending artificial intelligence with predictive maintenance tools and intelligence mapping technology such as Microsoft Enterprise BING Maps solution offers even more possibilities.
Hire expert developers for your next project
Imagine for a minute being able to read a trucking route from BING Maps that contains road attributes such as hill gradient, travel distance, and other such attributes, then blending asset maintenance datasets with mapping datasets to determine if a truck may or may not develop a fault during a long-haul trip.
These types of scenarios aren‘t outwith the realm of possibility as the datasets exist and can be fed into a machine learning model to arrive at predictions considering road, weather, and PdM data.
Simularity
We‘ve discussed a lot of the areas in which artificial intelligence can be used in conjunction with predictive maintenance, a firm that has invested heavily in AI within the context of PdM is Simularity.
The tech firm has developed a PdM solution that can be used in a wider number of sectors that include, but are not limited to healthcare, oil and gas, defense and the financial and insurance markets.
The solution can ingest more than one million data points per second and arrive at predictive scoring in just a few milliseconds. It uses complex machine learning models to achieve this and can also identify “event signatures” for certain events to help provide human operators with some context and defined maintenance instructions.
The solution can learn “on the fly” which means it doesn‘t have to be trained or deployed, can work almost “out of the box” and can run on devices as small as the Arduino or even be scaled out to run on multiple servers.
With such a wide number of use cases and almost limitless scalability on offer, AI-powered PdM solutions like Simularity are a real game-changer for enterprises.
For example, research by Simularity has identified that the cost of equipment maintenance and the resulting associated cost of failing equipment can be drastically reduced by deploying their PdM solution. The study went on to identify that, within the context of oil and gas, substantial cost savings can be made by:
- Reducing the downtime of oil wells due to logistics planning.
- Reducing the cost of damage to oil wells themselves.
- Reducing the cost of equipment needed to be replaced.
This is just one example of how products developed with artificial intelligence can be applied to the PdM field and it’s clear there are many benefits.
Interested in Predictive Maintenance AI for your Business?
In this blog post, we‘ve discussed how traditional, or preventive maintenance approaches can fall short of the mark and how AI with predictive maintenance can give businesses the edge when it comes to maintaining their existing hardware or infrastructure.
We‘ve seen how data science and AI-powered solutions can give the enterprise actionable insights and even recommend to engineers which components to replace — “just in time”.
If you are looking for an experienced team of data scientists and software developers to help you develop AI solutions for a predictive maintenance program, DevTeam.Space can help you.
You can easily get in touch via this quick form describing your initial requirements for using AI for predictive maintenance solutions. One of our technical managers will get back to you to answer your questions and connect you with relevant AI developers.
Frequently Asked Questions on Predictive Maintenance
They are corrective, preventive, risk-based, and condition-based maintenance.
Predictive maintenance is a complex process that requires a lot of time and training to implement. Staff needs to be trained in how to understand the data in order for this approach to be successful.
It has a number of key advantages, including lowering operating costs, improving productivity, reducing equipment wear and tear, and reducing the downtime caused by equipment failures.