Interested in knowing how to use AI to improve quality control? In this blog post, aimed at developers, CTOs, and business leaders, we look at information systems, how AI can be used in a planning and production context, and how developments in AI technology have led to a 4th industrial revolution.
For reference, following are the main areas that we will cover.
In this article
- How to Use AI to Improve Quality Control in Different Industries
- AI Robots: the 4th Industrial Revolution
- AI to Improve Quality Control and Wine Production
- Defect Recognition with IBM Watson
- Artificial Neural Networks for Quality Control
- Frequently Asked Questions on Using AI to Improve Quality Control
Artificial intelligence is everywhere. Futurologists, scientists, entrepreneurs, editors of popular scientific journals, ordinary citizens, and the public, all talk about it.
According to a study, “AI is poised to reach over US$116.4 billion by the year 2025, Deep Learning will bring in healthy gains adding significant momentum to global growth”.
However, it’s worth mentioning that despite having a plethora of amazing benefits, this advanced tech also has some downsides, so it’s a good idea to read more about its pros and cons.
We will now explore how to use AI to improve quality control in a variety of industries, including information systems, planning, and resource managment.
How to Use AI to Improve Quality Control in Different Industries
Information systems are a key factor for a business to be successful and help to ensure that quality assurance levels are being met in businesses, manufacturing plants, production lines, and supply chain processes.
It can‘t be denied, the rapid development of the software system over the past few decades has helped increase productivity and business efficiencies.
Integrated information systems allow businesses to monitor all data relevant to the business in real-time. This data include, but is not limited to:
- Preparatory data,
- Manufacturing data,
- Service process data,
- Asset management data,
- Material and resource management data,
- and product management data.
All of which helps businesses lower production and quality control costs. With profit margins being a core KPI, one can understand why introducing artificial intelligence components to existing information systems is a direction businesses are looking to take.
Resource Management
For example, consider the resource management of materials, tools, and documentation. This practice aims to ensure the right production processes yield the best cost for said resources.
A solution, powered by artificial intelligence, is a natural fit for such a use case.
Get a complimentary discovery call and a free ballpark estimate for your project
Trusted by 100x of startups and companies like
AI can be trained with historical entities, attributes, processes, and decisions to produce decision trees with the most optimal planning and production processes which can then be presented for humans to execute.
Another example may be the generation of a Bill of Materials (BOM) which details each component for a given product that must be manufactured.
AI can generate the most optimal steps required to produce the product whilst adhering to strict manufacturing industry standards.
Production Control, Planning and Strategy
There are three basic modules of planning and production control which are:
- MSPLAN (Master Schedule Plan)
- MRPLAN (Material Requirement Planning)
- CRPLAN (Capacity Requirements Planning and Scheduling)
A functional production system needs to blend all three modules holistically to ensure successful manufacturing and quality control.
Many difficulties in achieving production plans are due to insufficient representation of activities that are not part of the production process but are closely related to it.
Understanding this, and with existing knowledge, new principles such as DIKW (Data, Information, Knowledge, Wisdom) can be adopted.
DIKW is the logical structure of data, information, knowledge, and wisdom completing information hierarchy where each level adds certain properties above and below the previous one.
Data is the basic level, information gives context, knowledge adds to its use, and wisdom adds when and why to use it. The DIKW model assumes:
- The data is in the form of unforeseen observation and dimension,
- Information is formed by the analysis of connections and relationship between data,
- Knowledge is formed using information for action
- Wisdom is shaped using knowledge.
Structuring data in this logical fashion paves the way for businesses to implement “expert systems“ that go beyond traditional data manipulation and LOB (line of business) applications.
Experts, typically in STEM disciplines, program expert systems with the specifics of their problem domain (data, processes, entities, and attributes).
Thanks to their computation speeds and precision, the expert system is then able to surface actionable data that a human might have missed such as manufacturing anomalies.
An AI system is a departure from statically programmed expert systems and can be trained with historical data to make decisions by itself with no further programming.
We‘ll look at some examples of how artificial intelligence is being applied to help optimize the production process and how businesses are using AI to improve quality control procedures.
AI Robots: the 4th Industrial Revolution
After several decades of development, today’s robots and AI systems are the leaders of the Industry 4.0 concept.
They run home-based businesses, manage home appliances, connect with smartphones, provide information at stations, airports, and tourist zones, used as a part of traffic monitoring systems marking almost all kinds of vehicles, and they are capable to deliver packages.
Moreover, they are almost completely autonomous.
The use of robots being implemented in manufacturing positions is gradually increasing, and with more companies deciding to take such a move, this means fewer workplaces for humans.
Understandably, this is causing some concern among the human population and it is estimated that tens of millions of jobs will be lost due to automation implementation in manufacturing processes.
Considering that robots and AI systems significantly save financial resources and work faster and more efficiently than humans, we can say that the “right to work” for humans could be jeopardized.
Moreover, their production is growing, due to the increasing number of uses, but also because of the demand.
China is the world’s largest robot maker and their industry is increasingly being based on robots. The robot market industry is expected to reach $176.8 billion by 2025.
Elon Musk and other successful entrepreneurs have recently spoken of the need for universal income to help mitigate such issues in the future.
AI to Improve Quality Control and Wine Production
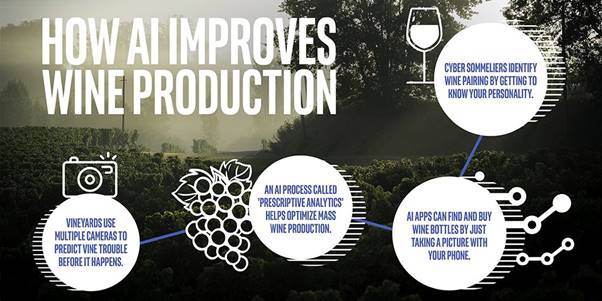
Hire expert developers for your next project
1,200 top developers
us since 2016
Artificial intelligence has the ability to deal with new situations, data, and circumstances that have not originally been anticipated.
When we add experience learning, which is enhanced by the constant repetition of tasks and performance measurement (machine learning), we get an almost autonomous system.
This is something that is increasingly being deployed in robotics and in the automotive industry, in the manufacturing process itself, but also in HR departments, wine production, pharmaceutical industry and various service sectors.
The purpose, among others, is of achieving considerable cost savings through performance targeted consumption of energy and other resources and enhanced quality control.
Wine, as it seems, in the future will be made by AI systems. Australian company, Ailytic, has developed an AI-powered system that will help large wine companies to optimize their production process.
The technique used in production optimization has been called “prescriptive analytics”. The result is a complete automation of temperature control, inventories and many other aspects of wine production.
Based on the set parameters, the program creates the best possible layout of the production steps which helps drive business savings.
Defect Recognition with IBM Watson
IBM is no stranger to AI and the IoT (Internet of Things). The tech giant built a solution around their Watson platform that provides a service that can inspect physical products.
The idea was to provide manufacturers with “cognitive assistants” in order to reduce errors and maximize product quality through computer vision-powered inspection tasks.
At an early stage of production, during testing, Watson shortened the validation time by 80 percent, and errors in manufacturing fell by 7 to 10 percent.
There are several components in the solution, you define the range of “training” images and store them in Watson. Watson then concludes whether the new products are “good” or “defective” using its training data.
The machine vision system uses an ultrasonic resolution camera that captures the products while they are moving through production and assembly. Together with human inspectors, Watson then recognizes defects in products, including scratches and damage.
The system can be set up on different production lines, can be trained onsite, and also auto-suggest changes which make the system smarter and further develops the inspection process.
This type of technology can be applied to a variety of industries, including the electronics and automotive industries, said Bret Greenstein, former IBM Global VP for IoT.
Reduced Downtime and Increased Productivity
According to IBM data, more than half of product quality checks include some form of visual confirmation. The visual inspection ensures that all parts are in the correct position, have the right shape, color, and texture, and have no scratches, holes or side particles.
Automation of visual inspection is difficult to accomplish due to the quantity and variety of products. Especially if one considers that the mistakes can be of any sort and size.
The system continually learns based on human evaluation and validation to spot mistakes in the images. The tool is designed to help manufacturers achieve the level of specialization they could not achieve so far manually.
It helps greatly in improving the quality control system, reducing downtime, and increasing productivity while delivering quality products.
You can find out more about IBMs IoT projects here.
Predictive Maintenance
Siemens sees predictive maintenance as being another key area where businesses can use AI to improve quality control while saving costs.
Predictive maintenance algorithms can monitor components at given timeframes, based on configurable parameters and components attributes.
Machine Learning algorithms and computer vision technology can then notify humans when the algorithm is 80% certain that a critical component will fail within the next 12 hours, all of which can help improve the quality of a manufacturing process.
You can find out more about Siemens Digital Factory Division here.
Hire expert developers for your next project
Artificial Neural Networks for Quality Control
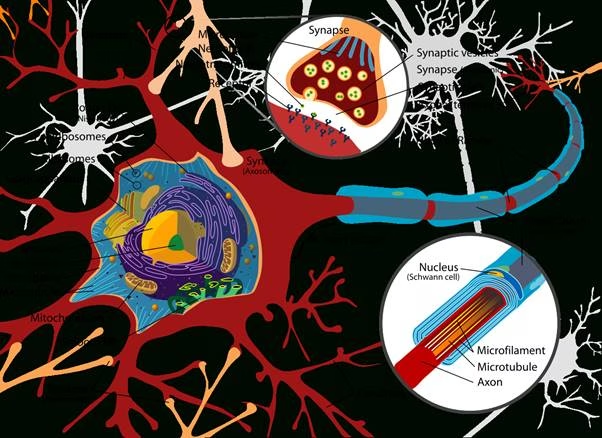
An artificial neural network, in its simplest explanation, is a powerful machine learning model that can be used to solve complex business problems.
The model is an attempt by computer scientists to mirror the structural models of the human brain, almost capable of processing nonlinear relationships between multiple sets of inputs and outputs.
Neural networks can make multiple decisions in parallel (whilst taking all data points into account) just like a human brain does.
In the case of quality control, a key advantage of neural networks is their flexibility or the possibility of finding adequate solutions based on incomplete data.
Artificial neural networks can also learn from examples and training data sets so they can quickly crack problems that are often difficult to solve by traditional software programs.
Tasks that were once performed by humans can now be passed onto a neural network for processing, thereby freeing up the workforce to focus on other creative activities that machines still struggle with.
A single trained network can also successfully control a production system in conditions where problems arise, again, relieving the workforce of mundane and manual maintenance tasks.
These types of artificial intelligence solutions can be found in the petroleum industry, for distribution of electricity, and are even being tested for airplane control.
Some applications of neural networks include, within the context of manufacturing, but are not limited to the following:
- Process control,
- Product design and analysis,
- Quality inspection systems,
- Machine maintenance analysis.
What Will Quality Control Look Like in the Future?
Foxconn, a technology manufacturer, is often contacted by various firms to assemble their products, some of their clients include businesses such as Apple and Microsoft.
Back in 2015, in a bid to improve working conditions for its human workforce, the firm made a commitment to introduce digitization by deploying 10,000 industrial robots to help deal with the ever-growing manufacturing demands for popular products such as iPhone and Xbox.
According to Foxconn, the digital transformation process has been slow, and whilst automating simple repetitive tasks is one thing, introducing systems that leverage AI is a different thing altogether.
The firm found that the AI system still does not have the cognitive ability of a human worker who is much better in the final phases of quality control and aesthetics checking of products.
It stands to reason that in the future, whilst some jobs may be lost to automation, others will be created as human capabilities are augmented with the arrival of AI-powered solutions.
Summary
In this blog post, we’ve explored information systems and how coupling advancements in software development and expert systems with production and planning strategy have led to the creation of cognitive computing initiatives.
These advanced systems greatly improve the manufacturing and quality control process.
Besides the huge sums to be made, innovating in this industry presents a chance to make a positive impact on people’s quality of life. Here’re a few amazing case studies of companies who hired DevTeam.Space to build their software products:
- Natural Language Processing for a Book Publisher — a natural language processing engine to classify ebooks by genre and customize social ads
- Face, Sex, Age, Recognition System — Machine Learning Program
- Hit Factor — Machine Learning Image Recognition App
If you’re looking to develop an AI-powered software solution and need assistance of a professional software development company, you can contact DevTeam.Space via this short specification form. One of our tech account managers experienced in handling AI projects will get back to you shortly to discuss the details.
Frequently Asked Questions on Using AI to Improve Quality Control
All systems can be improved by integrating new technologies and approaches. In the case of using AI to improve quality control, the implementation of AI and blockchain supply chain solutions will dramatically boost production and service quality.
We can improve AI by constantly developing and refining the code it is built on. As developers continue to improve the underlying code, AI systems will themselves be improving as they learn from their past results and errors.
The vast majority of the process that we all rely on today can be improved using AI. One day, AI will be capable of very advanced mathematics which will allow it to solve problems that have bewildered humans for years.